Receiving the following non descript error. Unforutantely, doesnt even let the backtest finish so I can further troubleshoot. Any idea how I might go about troubleshotting this? Theres a few things going on here, but high level:
KEI econmic indiciators dictates where in the cycle and what sectors should be getting bought. Algo goes forward and buys minimal shares of that sector ticker. Then depending on which sector ticker has active holdings, the algo looks at the universe pool of stocks, applies some TA to dictate buy and sells. Heres the error I am getting:
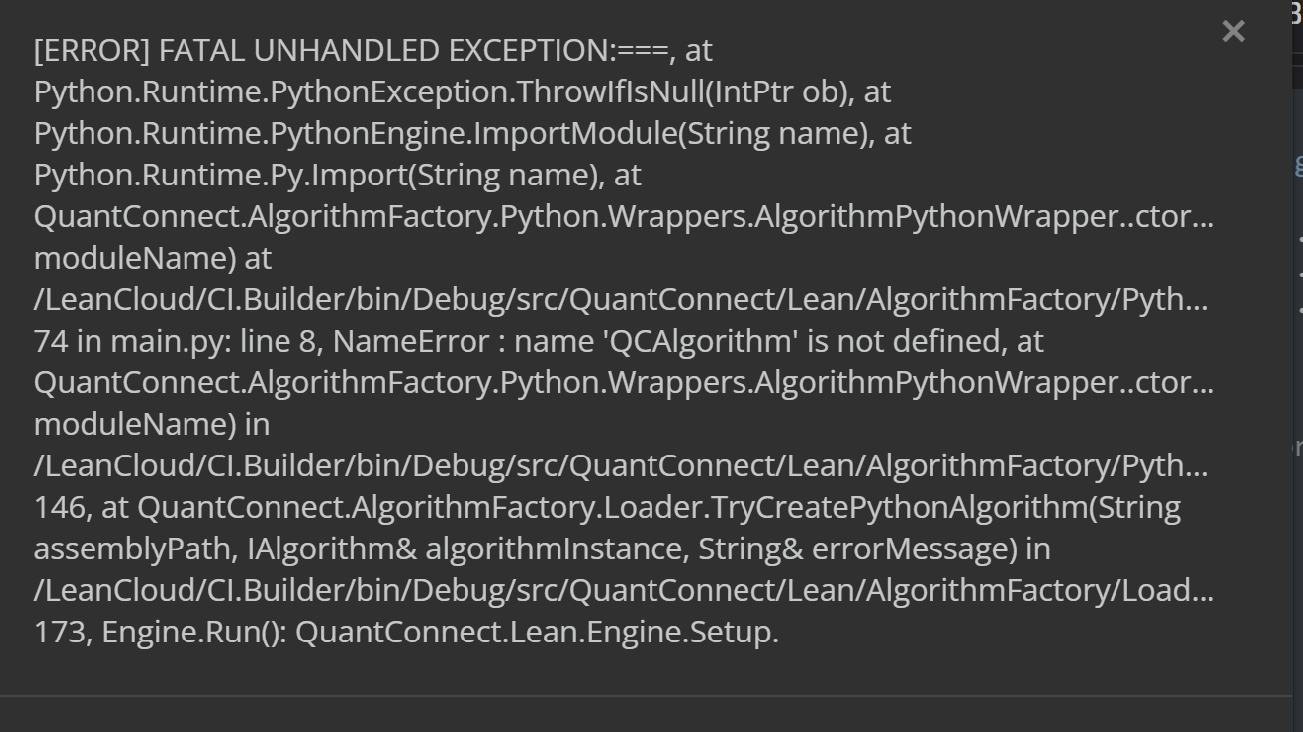
Heres the algo…
#from QuantConnect.Python import PythonQuandl
import numpy as np
import pandas as pd
import scipy as sc
from datetime import timedelta
from QuantConnect.Data.UniverseSelection import *
from Selection.FundamentalUniverseSelectionModel import FundamentalUniverseSelectionModel
class SmoothRedWhale(QCAlgorithm):
def Initialize(self):
self.SetStartDate(2022, 1, 1) # Set Start Date
#self.SetEndDate(2019, 1, 1)
self.SetCash(100000) # Set Strategy Cash
quandl_symbol = "OECD/KEI_LOLITOAA_OECDE_ST_M"
## Optional argument - personal token necessary for restricted dataset
#Quandl.SetAuthCode("PrzwuZR28Wqegvv1sdJ7")
self.SetBrokerageModel(BrokerageName.AlphaStreams)
self.SetWarmup(200)
self.init = True
self.kei = self.AddData(NasdaqDataLink, quandl_symbol, Resolution.Daily).Symbol
self.sma = self.SMA(self.kei, 1)
self.mom = self.MOMP(self.kei, 2)
self.XLFsector_symbolDataBySymbol = {}
self.XLEsector_symbolDataBySymbol = {}
self.XLBsector_symbolDataBySymbol = {}
self.XLIsector_symbolDataBySymbol = {}
self.XLYsector_symbolDataBySymbol = {}
self.XLPsector_symbolDataBySymbol = {}
self.XLUsector_symbolDataBySymbol = {}
self.XLKsector_symbolDataBySymbol = {}
self.XLVsector_symbolDataBySymbol = {}
self.XLCsector_symbolDataBySymbol = {}
self.XLEstocks = []
self.XLFstocks = []
self.XLBstocks = []
self.XLIstocks = []
self.XLYstocks = []
self.XLPstocks = []
self.XLUstocks = []
self.XLKstocks = []
self.XLVstocks = []
self.XLCstocks = []
self.trade = True
self.stateData = { }
self.SPY = self.AddEquity('SPY', Resolution.Daily).Symbol
self.stock = self.AddEquity('QQQ', Resolution.Hour).Symbol
self.bond = self.AddEquity('TMF', Resolution.Hour).Symbol
self.vix = self.AddEquity('VIX', Resolution.Minute).Symbol
self.XLF = self.AddEquity('XLF', Resolution.Hour).Symbol
self.XLE = self.AddEquity('XLE', Resolution.Hour).Symbol
self.XLB = self.AddEquity('XLB', Resolution.Hour).Symbol
self.XLI = self.AddEquity('XLI', Resolution.Hour).Symbol
self.XLY = self.AddEquity('XLY', Resolution.Hour).Symbol
self.XLP = self.AddEquity('XLP', Resolution.Hour).Symbol
self.XLU = self.AddEquity('XLU', Resolution.Hour).Symbol
self.XLK = self.AddEquity('XLK', Resolution.Hour).Symbol
self.XLV = self.AddEquity('XLV', Resolution.Hour).Symbol
self.XLC = self.AddEquity('XLC', Resolution.Hour).Symbol
#self.manually_selected = [self.SPY, self.stock, self.bond, self.vix, self.XLF, self.XLE, self.XLB, self.XLI, self.XLY, self.XLP, self.XLU, self.XLK, self.XLV, self.XLC]
self.manually_selected = []
# Stock Selector
self.UniverseSettings.Resolution = Resolution.Minute
self.universeXLF = self.AddUniverse(self.coarse, self.XLFfine)
self.universeXLE = self.AddUniverse(self.coarse, self.XLEfine)
self.universeXLB = self.AddUniverse(self.coarse, self.XLBfine)
self.universeXLI = self.AddUniverse(self.coarse, self.XLIfine)
self.universeXLY = self.AddUniverse(self.coarse, self.XLYfine)
self.universeXLP = self.AddUniverse(self.coarse, self.XLPfine)
self.universeXLU = self.AddUniverse(self.coarse, self.XLUfine)
self.universeXLK = self.AddUniverse(self.coarse, self.XLKfine)
self.universeXLV = self.AddUniverse(self.coarse, self.XLVfine)
self.universeXLC = self.AddUniverse(self.coarse, self.XLCfine)
self.SetWarmup(500)
self.symbolBySectorCode = dict()
self.__numberOfSymbols = 1000
self.__numberOfSymbolsFine = 3
self.addedsymbols = [self.SPY, self.stock, self.bond, self.vix, self.XLF, self.XLE, self.XLB, self.XLI, self.XLY, self.XLP, self.XLU, self.XLK, self.XLV, self.XLC]
self.Schedule.On(self.DateRules.EveryDay(self.stock), self.TimeRules.AfterMarketOpen(self.stock, 1),
self.Rebalance)
self.Schedule.On(self.DateRules.EveryDay(self.stock), self.TimeRules.AfterMarketOpen(self.stock, 5),
self.Collectsymboldata)
#set the following between 1 - 4 hours depending on buy frequency
self.Schedule.On(self.DateRules.EveryDay(self.stock),
self.TimeRules.Every(timedelta(hours=1)),
self.buySignals)
# self.Schedule.On(self.DateRules.EveryDay(self.stock),
# self.TimeRules.Every(timedelta(hours=3.25)),
# self.sellSignals)
self.Schedule.On(self.DateRules.EveryDay("SPY"),
self.TimeRules.AfterMarketOpen("SPY"),
self.tradeStart)
self.Schedule.On(self.DateRules.EveryDay("SPY"),
self.TimeRules.BeforeMarketClose("SPY"),
self.tradeEnd)
def OnData(self, data):
#for symbol in self.activelyTrading:
# if not self.Portfolio[symbol].Invested:
# self.SetHoldings(symbol, self.weight)
# Note: in the below, x is Symbol and x.Value is string
self.Debug(f"XLU universe includes: {[x.Value for x in self.XLUstocks]}")
self.Debug(f"XLU has data on: {[x.Value for x in self.XLUsector_symbolDataBySymbol]}")
#self.Debug(f"Actively Trading: {[x.Value for x in self.activelyTrading]}")
# self.Debug(f"XLB universe includes: {[x.Value for x in self.XLBstocks]}")
# self.Debug(f"XLI universe includes: {[x.Value for x in self.XLIstocks]}")
# self.Debug(f"XLY universe includes: {[x.Value for x in self.XLYstocks]}")
# self.Debug(f"XLP universe includes: {[x.Value for x in self.XLPstocks]}")
# self.Debug(f"XLU universe includes: {[x.Value for x in self.XLUstocks]}")
# self.Debug(f"XLK universe includes: {[x.Value for x in self.XLKstocks]}")
# self.Debug(f"XLV universe includes: {[x.Value for x in self.XLVstocks]}")
# self.Debug(f"XLC universe includes: {[x.Value for x in self.XLCstocks]}")
def tradeStart(self):
self.trade = True
def tradeEnd(self):
self.trade = False
def Collectsymboldata(self):
for symbol in self.XLFstocks:
self.AddEquity(symbol, Resolution.Hour)
ema10 = self.EMA(symbol, 10, Resolution.Hour, Field.Close)
sma200 = self.SMA(symbol, 200, Resolution.Daily, Field.Close)
sma7 = self.SMA(symbol, 7, Resolution.Hour, Field.Close)
sma20 = self.SMA(symbol, 20, Resolution.Daily, Field.Close)
sma50 = self.SMA(symbol, 50, Resolution.Daily, Field.Close)
ema20 = self.EMA(symbol, 20, Resolution.Hour, Field.Close)
ema50 = self.EMA(symbol, 50, Resolution.Hour, Field.Close)
rsi = self.RSI(symbol, 14, Resolution.Daily)
wilr = self.WILR(symbol, 14, Resolution.Daily)
wilr_fast = self.WILR(symbol, 10, Resolution.Daily)
high = self.MAX(symbol, int(self.GetParameter("highHist")), Resolution.Daily, Field.High)
midhigh = self.MAX(symbol, 3, Resolution.Daily, Field.High)
low = self.MIN(symbol, int(self.GetParameter("lowHist")), Resolution.Daily, Field.Low)
stoplow = self.MIN(symbol, 20, Resolution.Daily, Field.Low)
symbolData = SymbolData(symbol, sma7, ema10, sma20, sma200, sma50, ema20, rsi, wilr, wilr_fast, high, midhigh, low, stoplow)
self.XLFsector_symbolDataBySymbol[symbol] = symbolData
for symbol in self.XLEstocks:
self.AddEquity(symbol, Resolution.Hour)
ema10 = self.EMA(symbol, 10, Resolution.Hour, Field.Close)
sma200 = self.SMA(symbol, 200, Resolution.Daily, Field.Close)
sma7 = self.SMA(symbol, 7, Resolution.Hour, Field.Close)
sma20 = self.SMA(symbol, 20, Resolution.Daily, Field.Close)
sma50 = self.SMA(symbol, 50, Resolution.Daily, Field.Close)
ema20 = self.EMA(symbol, 20, Resolution.Hour, Field.Close)
ema50 = self.EMA(symbol, 50, Resolution.Hour, Field.Close)
rsi = self.RSI(symbol, 14, Resolution.Daily)
wilr = self.WILR(symbol, 14, Resolution.Daily)
wilr_fast = self.WILR(symbol, 10, Resolution.Daily)
high = self.MAX(symbol, int(self.GetParameter("highHist")), Resolution.Daily, Field.High)
midhigh = self.MAX(symbol, 3, Resolution.Daily, Field.High)
low = self.MIN(symbol, int(self.GetParameter("lowHist")), Resolution.Daily, Field.Low)
stoplow = self.MIN(symbol, 20, Resolution.Daily, Field.Low)
symbolData = SymbolData(symbol, sma7, ema10, sma20, sma200, sma50, ema20, rsi, wilr, wilr_fast, high, midhigh, low, stoplow)
self.XLEsector_symbolDataBySymbol[symbol] = symbolData
for symbol in self.XLBstocks:
self.AddEquity(symbol, Resolution.Hour)
ema10 = self.EMA(symbol, 10, Resolution.Hour, Field.Close)
sma200 = self.SMA(symbol, 200, Resolution.Daily, Field.Close)
sma7 = self.SMA(symbol, 7, Resolution.Hour, Field.Close)
sma20 = self.SMA(symbol, 20, Resolution.Daily, Field.Close)
sma50 = self.SMA(symbol, 50, Resolution.Daily, Field.Close)
ema20 = self.EMA(symbol, 20, Resolution.Hour, Field.Close)
ema50 = self.EMA(symbol, 50, Resolution.Hour, Field.Close)
rsi = self.RSI(symbol, 14, Resolution.Daily)
wilr = self.WILR(symbol, 14, Resolution.Daily)
wilr_fast = self.WILR(symbol, 10, Resolution.Daily)
high = self.MAX(symbol, int(self.GetParameter("highHist")), Resolution.Daily, Field.High)
midhigh = self.MAX(symbol, 3, Resolution.Daily, Field.High)
low = self.MIN(symbol, int(self.GetParameter("lowHist")), Resolution.Daily, Field.Low)
stoplow = self.MIN(symbol, 20, Resolution.Daily, Field.Low)
symbolData = SymbolData(symbol, sma7, ema10, sma20, sma200, sma50, ema20, rsi, wilr, wilr_fast, high, midhigh, low, stoplow)
self.XLBsector_symbolDataBySymbol[symbol] = symbolData
for symbol in self.XLIstocks:
self.AddEquity(symbol, Resolution.Hour)
ema10 = self.EMA(symbol, 10, Resolution.Hour, Field.Close)
sma200 = self.SMA(symbol, 200, Resolution.Daily, Field.Close)
sma7 = self.SMA(symbol, 7, Resolution.Hour, Field.Close)
sma20 = self.SMA(symbol, 20, Resolution.Daily, Field.Close)
sma50 = self.SMA(symbol, 50, Resolution.Daily, Field.Close)
ema20 = self.EMA(symbol, 20, Resolution.Hour, Field.Close)
ema50 = self.EMA(symbol, 50, Resolution.Hour, Field.Close)
rsi = self.RSI(symbol, 14, Resolution.Daily)
wilr = self.WILR(symbol, 14, Resolution.Daily)
wilr_fast = self.WILR(symbol, 10, Resolution.Daily)
high = self.MAX(symbol, int(self.GetParameter("highHist")), Resolution.Daily, Field.High)
midhigh = self.MAX(symbol, 3, Resolution.Daily, Field.High)
low = self.MIN(symbol, int(self.GetParameter("lowHist")), Resolution.Daily, Field.Low)
stoplow = self.MIN(symbol, 20, Resolution.Daily, Field.Low)
symbolData = SymbolData(symbol, sma7, ema10, sma20, sma200, sma50, ema20, rsi, wilr, wilr_fast, high, midhigh, low, stoplow)
self.XLIsector_symbolDataBySymbol[symbol] = symbolData
for symbol in self.XLYstocks:
self.AddEquity(symbol, Resolution.Hour)
ema10 = self.EMA(symbol, 10, Resolution.Hour, Field.Close)
sma200 = self.SMA(symbol, 200, Resolution.Daily, Field.Close)
sma7 = self.SMA(symbol, 7, Resolution.Hour, Field.Close)
sma20 = self.SMA(symbol, 20, Resolution.Daily, Field.Close)
sma50 = self.SMA(symbol, 50, Resolution.Daily, Field.Close)
ema20 = self.EMA(symbol, 20, Resolution.Hour, Field.Close)
ema50 = self.EMA(symbol, 50, Resolution.Hour, Field.Close)
rsi = self.RSI(symbol, 14, Resolution.Daily)
wilr = self.WILR(symbol, 14, Resolution.Daily)
wilr_fast = self.WILR(symbol, 10, Resolution.Daily)
high = self.MAX(symbol, int(self.GetParameter("highHist")), Resolution.Daily, Field.High)
midhigh = self.MAX(symbol, 3, Resolution.Daily, Field.High)
low = self.MIN(symbol, int(self.GetParameter("lowHist")), Resolution.Daily, Field.Low)
stoplow = self.MIN(symbol, 20, Resolution.Daily, Field.Low)
symbolData = SymbolData(symbol, sma7, ema10, sma20, sma200, sma50, ema20, rsi, wilr, wilr_fast, high, midhigh, low, stoplow)
self.XLYsector_symbolDataBySymbol[symbol] = symbolData
for symbol in self.XLPstocks:
self.AddEquity(symbol, Resolution.Hour)
ema10 = self.EMA(symbol, 10, Resolution.Hour, Field.Close)
sma200 = self.SMA(symbol, 200, Resolution.Daily, Field.Close)
sma7 = self.SMA(symbol, 7, Resolution.Hour, Field.Close)
sma20 = self.SMA(symbol, 20, Resolution.Daily, Field.Close)
sma50 = self.SMA(symbol, 50, Resolution.Daily, Field.Close)
ema20 = self.EMA(symbol, 20, Resolution.Hour, Field.Close)
ema50 = self.EMA(symbol, 50, Resolution.Hour, Field.Close)
rsi = self.RSI(symbol, 14, Resolution.Daily)
wilr = self.WILR(symbol, 14, Resolution.Daily)
wilr_fast = self.WILR(symbol, 10, Resolution.Daily)
high = self.MAX(symbol, int(self.GetParameter("highHist")), Resolution.Daily, Field.High)
midhigh = self.MAX(symbol, 3, Resolution.Daily, Field.High)
low = self.MIN(symbol, int(self.GetParameter("lowHist")), Resolution.Daily, Field.Low)
stoplow = self.MIN(symbol, 20, Resolution.Daily, Field.Low)
symbolData = SymbolData(symbol, sma7, ema10, sma20, sma200, sma50, ema20, rsi, wilr, wilr_fast, high, midhigh, low, stoplow)
self.XLPsector_symbolDataBySymbol[symbol] = symbolData
for symbol in self.XLUstocks:
self.AddEquity(symbol, Resolution.Hour)
ema10 = self.EMA(symbol, 10, Resolution.Hour, Field.Close)
sma200 = self.SMA(symbol, 200, Resolution.Daily, Field.Close)
sma7 = self.SMA(symbol, 7, Resolution.Hour, Field.Close)
sma20 = self.SMA(symbol, 20, Resolution.Daily, Field.Close)
sma50 = self.SMA(symbol, 50, Resolution.Daily, Field.Close)
ema20 = self.EMA(symbol, 20, Resolution.Hour, Field.Close)
ema50 = self.EMA(symbol, 50, Resolution.Hour, Field.Close)
rsi = self.RSI(symbol, 14, Resolution.Daily)
wilr = self.WILR(symbol, 14, Resolution.Daily)
wilr_fast = self.WILR(symbol, 10, Resolution.Daily)
high = self.MAX(symbol, int(self.GetParameter("highHist")), Resolution.Daily, Field.High)
midhigh = self.MAX(symbol, 3, Resolution.Daily, Field.High)
low = self.MIN(symbol, int(self.GetParameter("lowHist")), Resolution.Daily, Field.Low)
stoplow = self.MIN(symbol, 20, Resolution.Daily, Field.Low)
symbolData = SymbolData(symbol, sma7, ema10, sma20, sma200, sma50, ema20, rsi, wilr, wilr_fast, high, midhigh, low, stoplow)
self.XLUsector_symbolDataBySymbol[symbol] = symbolData
for symbol in self.XLKstocks:
self.AddEquity(symbol, Resolution.Hour)
ema10 = self.EMA(symbol, 10, Resolution.Hour, Field.Close)
sma200 = self.SMA(symbol, 200, Resolution.Daily, Field.Close)
sma7 = self.SMA(symbol, 7, Resolution.Hour, Field.Close)
sma20 = self.SMA(symbol, 20, Resolution.Daily, Field.Close)
sma50 = self.SMA(symbol, 50, Resolution.Daily, Field.Close)
ema20 = self.EMA(symbol, 20, Resolution.Hour, Field.Close)
ema50 = self.EMA(symbol, 50, Resolution.Hour, Field.Close)
rsi = self.RSI(symbol, 14, Resolution.Daily)
wilr = self.WILR(symbol, 14, Resolution.Daily)
wilr_fast = self.WILR(symbol, 10, Resolution.Daily)
high = self.MAX(symbol, int(self.GetParameter("highHist")), Resolution.Daily, Field.High)
midhigh = self.MAX(symbol, 3, Resolution.Daily, Field.High)
low = self.MIN(symbol, int(self.GetParameter("lowHist")), Resolution.Daily, Field.Low)
stoplow = self.MIN(symbol, 20, Resolution.Daily, Field.Low)
symbolData = SymbolData(symbol, sma7, ema10, sma20, sma200, sma50, ema20, rsi, wilr, wilr_fast, high, midhigh, low, stoplow)
self.XLKsector_symbolDataBySymbol[symbol] = symbolData
def coarse(self, coarse):
for i in coarse:
if i.Symbol not in self.stateData:
self.stateData[i.Symbol] = SelectionData(i.Symbol, 200)
# Updates the SymbolData object with current EOD price
avg = self.stateData[i.Symbol]
avg.update(i.EndTime, i.AdjustedPrice, i.DollarVolume)
# Filter the values of the dict to those above EMA and more than $1B vol.
# CoarseWithFundamental = [x for x in self.stateData.values() if x.is_above_ema and x.volume > 1000000000]
# sort by the largest in volume.
# values.sort(key=lambda x: x.volume, reverse=True)
# we need to return only the symbol objects
# self.coarselist = [ x.symbol for x in values[:self.__numberOfSymbols] ]
#CoarseWithFundamental = [x for x in coarse if (x.HasFundamentalData) and (float(x.Price) > 10)]
#sortedByDollarVolume = sorted(CoarseWithFundamental, key=lambda x: x.DollarVolume, reverse=True)
#top = sortedByDollarVolume[:self.__numberOfSymbols]
#
# self.coarselist = [i.Symbol for i in top]
# for symbol in self.manually_selected:
# self.coarselist.append(symbol)
##################
# We are going to use a dictionary to refer the object that will keep the moving averages
for cf in coarse:
if cf.Symbol not in self.averages:
self.averages[cf.Symbol] = SymbolData(cf.Symbol)
# Updates the SymbolData object with current EOD price
avg = self.averages[cf.Symbol]
avg.update(cf.EndTime, cf.AdjustedPrice)
# Filter the values of the dict: we only want up-trending securities
values = list(filter(lambda x: x.is_uptrend, self.averages.values()))
# Sorts the values of the dict: we want those with greater difference between the moving averages
values.sort(key=lambda x: x.scale, reverse=True)
for x in values[:self.__numberOfSymbols]:
self.Log('symbol: ' + str(x.symbol.Value) + ' scale: ' + str(x.scale))
# we need to return only the symbol objects
return [ x.symbol for x in values[:self.__numberOfSymbols] ]
#
# CoarseWithFundamental = [x for x in coarse if (x.HasFundamentalData) and x.is_above_ema and (float(x.Price) > 5)]
# sortedByDollarVolume = sorted(CoarseWithFundamental, key=lambda x: x.DollarVolume, reverse=True) 1
# top = sortedByDollarVolume[:self.__numberOfSymbols]
# self.coarselist = [i.Symbol for i in top]
return self.coarselist
def XLFfine(self, fine):
filtered_fine = [x for x in fine if x.AssetClassification.MorningstarSectorCode == MorningstarSectorCode.FinancialServices
and (x.ValuationRatios.EVToEBITDA > 0)
and (x.EarningReports.BasicAverageShares.ThreeMonths > 0)
and x.EarningReports.BasicAverageShares.ThreeMonths * (x.EarningReports.BasicEPS.TwelveMonths*x.ValuationRatios.PERatio) > 2e9]
sortedByfactor1 = sorted(filtered_fine, key=lambda x: x.OperationRatios.ROIC.Value, reverse=True)
sortedByfactor2 = sorted(filtered_fine, key=lambda x: x.OperationRatios.LongTermDebtEquityRatio.Value, reverse=True)
sortedByfactor3 = sorted(filtered_fine, key=lambda x: x.ValuationRatios.FCFYield, reverse=True)
stock_dict = {}
# assign a score to each stock, you can also change the rule of scoring here.
for i,ele in enumerate(sortedByfactor1):
rank1 = i
rank2 = sortedByfactor2.index(ele)
rank3 = sortedByfactor3.index(ele)
score = sum([rank1*0.4,rank2*0.2,rank3*0.4])
stock_dict[ele] = score
# sort the stocks by their scores
self.sorted_stock = sorted(stock_dict.items(), key=lambda d:d[1],reverse=False)
sorted_symbol = [x[0] for x in self.sorted_stock]
self.sorted_stock = sorted(stock_dict.items(), key=lambda d:d[1],reverse=True)
#
self.sorted_symbol = [self.sorted_stock[i][0] for i in range(len(self.sorted_stock))]
topFine = self.sorted_symbol[:self.__numberOfSymbolsFine]
#topFine = sortedByNormalizedPE[:self.__numberOfSymbolsFine]
self.XLFstocks = [i.Symbol for i in topFine]
#for symbol in self.manually_selected:
# self.XLFstocks.append(symbol)
return self.XLFstocks
def XLEfine(self, fine):
filtered_fine = [x for x in fine if x.AssetClassification.MorningstarSectorCode == MorningstarSectorCode.Energy
and (x.ValuationRatios.EVToEBITDA > 0)
and (x.EarningReports.BasicAverageShares.ThreeMonths > 0)
and x.EarningReports.BasicAverageShares.ThreeMonths * (x.EarningReports.BasicEPS.TwelveMonths*x.ValuationRatios.PERatio) > 2e9]
sortedByfactor1 = sorted(filtered_fine, key=lambda x: x.OperationRatios.ROIC.Value, reverse=True)
sortedByfactor2 = sorted(filtered_fine, key=lambda x: x.OperationRatios.LongTermDebtEquityRatio.Value, reverse=True)
sortedByfactor3 = sorted(filtered_fine, key=lambda x: x.ValuationRatios.FCFYield, reverse=True)
stock_dict = {}
# assign a score to each stock, you can also change the rule of scoring here.
for i,ele in enumerate(sortedByfactor1):
rank1 = i
rank2 = sortedByfactor2.index(ele)
rank3 = sortedByfactor3.index(ele)
score = sum([rank1*0.4,rank2*0.2,rank3*0.4])
stock_dict[ele] = score
# sort the stocks by their scores
self.sorted_stock = sorted(stock_dict.items(), key=lambda d:d[1],reverse=False)
sorted_symbol = [x[0] for x in self.sorted_stock]
self.sorted_stock = sorted(stock_dict.items(), key=lambda d:d[1],reverse=True)
#
self.sorted_symbol = [self.sorted_stock[i][0] for i in range(len(self.sorted_stock))]
topFine = self.sorted_symbol[:self.__numberOfSymbolsFine]
self.XLEstocks = [i.Symbol for i in topFine]
# for symbol in self.manually_selected:
# self.XLEstocks.append(symbol)
return self.XLEstocks
def XLBfine(self, fine):
filtered_fine = [x for x in fine if x.AssetClassification.MorningstarSectorCode == MorningstarSectorCode.BasicMaterials
and (x.ValuationRatios.EVToEBITDA > 0)
and (x.EarningReports.BasicAverageShares.ThreeMonths > 0)
and x.EarningReports.BasicAverageShares.ThreeMonths * (x.EarningReports.BasicEPS.TwelveMonths*x.ValuationRatios.PERatio) > 2e9]
sortedByfactor1 = sorted(filtered_fine, key=lambda x: x.OperationRatios.ROIC.Value, reverse=True)
sortedByfactor2 = sorted(filtered_fine, key=lambda x: x.OperationRatios.LongTermDebtEquityRatio.Value, reverse=True)
sortedByfactor3 = sorted(filtered_fine, key=lambda x: x.ValuationRatios.FCFYield, reverse=True)
stock_dict = {}
# assign a score to each stock, you can also change the rule of scoring here.
for i,ele in enumerate(sortedByfactor1):
rank1 = i
rank2 = sortedByfactor2.index(ele)
rank3 = sortedByfactor3.index(ele)
score = sum([rank1*0.4,rank2*0.2,rank3*0.4])
stock_dict[ele] = score
# sort the stocks by their scores
self.sorted_stock = sorted(stock_dict.items(), key=lambda d:d[1],reverse=False)
sorted_symbol = [x[0] for x in self.sorted_stock]
self.sorted_stock = sorted(stock_dict.items(), key=lambda d:d[1],reverse=True)
#
self.sorted_symbol = [self.sorted_stock[i][0] for i in range(len(self.sorted_stock))]
topFine = self.sorted_symbol[:self.__numberOfSymbolsFine]
self.XLBstocks = [i.Symbol for i in topFine]
# for symbol in self.manually_selected:
# self.XLBstocks.append(symbol)
return self.XLBstocks
def XLIfine(self, fine):
filtered_fine = [x for x in fine if x.AssetClassification.MorningstarSectorCode == MorningstarSectorCode.Industrials
and (x.ValuationRatios.EVToEBITDA > 0)
and (x.EarningReports.BasicAverageShares.ThreeMonths > 0)
and x.EarningReports.BasicAverageShares.ThreeMonths * (x.EarningReports.BasicEPS.TwelveMonths*x.ValuationRatios.PERatio) > 2e9]
sortedByfactor1 = sorted(filtered_fine, key=lambda x: x.OperationRatios.ROIC.Value, reverse=True)
sortedByfactor2 = sorted(filtered_fine, key=lambda x: x.OperationRatios.LongTermDebtEquityRatio.Value, reverse=True)
sortedByfactor3 = sorted(filtered_fine, key=lambda x: x.ValuationRatios.FCFYield, reverse=True)
stock_dict = {}
# assign a score to each stock, you can also change the rule of scoring here.
for i,ele in enumerate(sortedByfactor1):
rank1 = i
rank2 = sortedByfactor2.index(ele)
rank3 = sortedByfactor3.index(ele)
score = sum([rank1*0.4,rank2*0.2,rank3*0.4])
stock_dict[ele] = score
# sort the stocks by their scores
self.sorted_stock = sorted(stock_dict.items(), key=lambda d:d[1],reverse=False)
sorted_symbol = [x[0] for x in self.sorted_stock]
self.sorted_stock = sorted(stock_dict.items(), key=lambda d:d[1],reverse=True)
#
self.sorted_symbol = [self.sorted_stock[i][0] for i in range(len(self.sorted_stock))]
topFine = self.sorted_symbol[:self.__numberOfSymbolsFine]
self.XLIstocks = [i.Symbol for i in topFine]
# for symbol in self.manually_selected:
# self.XLIstocks.append(symbol)
return self.XLIstocks
def XLYfine(self, fine):
filtered_fine = [x for x in fine if x.AssetClassification.MorningstarSectorCode == MorningstarSectorCode.ConsumerCyclical
and (x.ValuationRatios.EVToEBITDA > 0)
and (x.EarningReports.BasicAverageShares.ThreeMonths > 0)
and x.EarningReports.BasicAverageShares.ThreeMonths * (x.EarningReports.BasicEPS.TwelveMonths*x.ValuationRatios.PERatio) > 2e9]
sortedByfactor1 = sorted(filtered_fine, key=lambda x: x.OperationRatios.ROIC.Value, reverse=True)
sortedByfactor2 = sorted(filtered_fine, key=lambda x: x.OperationRatios.LongTermDebtEquityRatio.Value, reverse=True)
sortedByfactor3 = sorted(filtered_fine, key=lambda x: x.ValuationRatios.FCFYield, reverse=True)
stock_dict = {}
# assign a score to each stock, you can also change the rule of scoring here.
for i,ele in enumerate(sortedByfactor1):
rank1 = i
rank2 = sortedByfactor2.index(ele)
rank3 = sortedByfactor3.index(ele)
score = sum([rank1*0.4,rank2*0.2,rank3*0.4])
stock_dict[ele] = score
# sort the stocks by their scores
self.sorted_stock = sorted(stock_dict.items(), key=lambda d:d[1],reverse=False)
sorted_symbol = [x[0] for x in self.sorted_stock]
self.sorted_stock = sorted(stock_dict.items(), key=lambda d:d[1],reverse=True)
#
self.sorted_symbol = [self.sorted_stock[i][0] for i in range(len(self.sorted_stock))]
topFine = self.sorted_symbol[:self.__numberOfSymbolsFine]
self.XLYstocks = [i.Symbol for i in topFine]
# for symbol in self.manually_selected:
# self.XLYstocks.append(symbol)
return self.XLYstocks
def XLPfine(self, fine):
filtered_fine = [x for x in fine if x.AssetClassification.MorningstarSectorCode == MorningstarSectorCode.ConsumerDefensive
and (x.ValuationRatios.EVToEBITDA > 0)
and (x.EarningReports.BasicAverageShares.ThreeMonths > 0)
and x.EarningReports.BasicAverageShares.ThreeMonths * (x.EarningReports.BasicEPS.TwelveMonths*x.ValuationRatios.PERatio) > 2e9]
sortedByfactor1 = sorted(filtered_fine, key=lambda x: x.OperationRatios.ROIC.Value, reverse=True)
sortedByfactor2 = sorted(filtered_fine, key=lambda x: x.OperationRatios.LongTermDebtEquityRatio.Value, reverse=True)
sortedByfactor3 = sorted(filtered_fine, key=lambda x: x.ValuationRatios.FCFYield, reverse=True)
stock_dict = {}
# assign a score to each stock, you can also change the rule of scoring here.
for i,ele in enumerate(sortedByfactor1):
rank1 = i
rank2 = sortedByfactor2.index(ele)
rank3 = sortedByfactor3.index(ele)
score = sum([rank1*0.4,rank2*0.2,rank3*0.4])
stock_dict[ele] = score
# sort the stocks by their scores
self.sorted_stock = sorted(stock_dict.items(), key=lambda d:d[1],reverse=False)
sorted_symbol = [x[0] for x in self.sorted_stock]
self.sorted_stock = sorted(stock_dict.items(), key=lambda d:d[1],reverse=True)
#
self.sorted_symbol = [self.sorted_stock[i][0] for i in range(len(self.sorted_stock))]
topFine = self.sorted_symbol[:self.__numberOfSymbolsFine]
self.XLPstocks = [i.Symbol for i in topFine]
# for symbol in self.manually_selected:
# self.XLPstocks.append(symbol)
return self.XLPstocks
def XLUfine(self, fine):
filtered_fine = [x for x in fine if x.AssetClassification.MorningstarSectorCode == MorningstarSectorCode.Utilities
]
sortedByfactor1 = sorted(filtered_fine, key=lambda x: x.OperationRatios.ROIC.Value, reverse=True)
sortedByfactor2 = sorted(filtered_fine, key=lambda x: x.OperationRatios.LongTermDebtEquityRatio.Value, reverse=True)
sortedByfactor3 = sorted(filtered_fine, key=lambda x: x.ValuationRatios.FCFYield, reverse=True)
stock_dict = {}
# assign a score to each stock, you can also change the rule of scoring here.
for i,ele in enumerate(sortedByfactor1):
rank1 = i
rank2 = sortedByfactor2.index(ele)
rank3 = sortedByfactor3.index(ele)
score = sum([rank1*0.4,rank2*0.2,rank3*0.4])
stock_dict[ele] = score
# sort the stocks by their scores
self.sorted_stock = sorted(stock_dict.items(), key=lambda d:d[1],reverse=False)
sorted_symbol = [x[0] for x in self.sorted_stock]
self.sorted_stock = sorted(stock_dict.items(), key=lambda d:d[1],reverse=True)
#
self.sorted_symbol = [self.sorted_stock[i][0] for i in range(len(self.sorted_stock))]
topFine = self.sorted_symbol[:self.__numberOfSymbolsFine]
self.XLUstocks = [i.Symbol for i in topFine]
# for symbol in self.manually_selected:
# self.XLUstocks.append(symbol)
return self.XLUstocks
def XLKfine(self, fine):
filtered_fine = [x for x in fine if x.AssetClassification.MorningstarSectorCode == MorningstarSectorCode.Technology
and (x.ValuationRatios.EVToEBITDA > 0)
and (x.EarningReports.BasicAverageShares.ThreeMonths > 0)
and x.EarningReports.BasicAverageShares.ThreeMonths * (x.EarningReports.BasicEPS.TwelveMonths*x.ValuationRatios.PERatio) > 2e9]
sortedByfactor1 = sorted(filtered_fine, key=lambda x: x.OperationRatios.ROIC.Value, reverse=True)
sortedByfactor2 = sorted(filtered_fine, key=lambda x: x.OperationRatios.LongTermDebtEquityRatio.Value, reverse=True)
sortedByfactor3 = sorted(filtered_fine, key=lambda x: x.ValuationRatios.FCFYield, reverse=True)
stock_dict = {}
# assign a score to each stock, you can also change the rule of scoring here.
for i,ele in enumerate(sortedByfactor1):
rank1 = i
rank2 = sortedByfactor2.index(ele)
rank3 = sortedByfactor3.index(ele)
score = sum([rank1*0.4,rank2*0.2,rank3*0.4])
stock_dict[ele] = score
# sort the stocks by their scores
self.sorted_stock = sorted(stock_dict.items(), key=lambda d:d[1],reverse=False)
sorted_symbol = [x[0] for x in self.sorted_stock]
self.sorted_stock = sorted(stock_dict.items(), key=lambda d:d[1],reverse=True)
#
self.sorted_symbol = [self.sorted_stock[i][0] for i in range(len(self.sorted_stock))]
topFine = self.sorted_symbol[:self.__numberOfSymbolsFine]
self.XLKstocks = [i.Symbol for i in topFine]
# for symbol in self.manually_selected:
# self.XLKstocks.append(symbol)
return self.XLKstocks
def XLVfine(self, fine):
filtered_fine = [x for x in fine if x.AssetClassification.MorningstarSectorCode == MorningstarSectorCode.Healthcare
and (x.ValuationRatios.EVToEBITDA > 0)
and (x.EarningReports.BasicAverageShares.ThreeMonths > 0)
and x.EarningReports.BasicAverageShares.ThreeMonths * (x.EarningReports.BasicEPS.TwelveMonths*x.ValuationRatios.PERatio) > 2e9]
sortedByfactor1 = sorted(filtered_fine, key=lambda x: x.OperationRatios.ROIC.Value, reverse=True)
sortedByfactor2 = sorted(filtered_fine, key=lambda x: x.OperationRatios.LongTermDebtEquityRatio.Value, reverse=True)
sortedByfactor3 = sorted(filtered_fine, key=lambda x: x.ValuationRatios.FCFYield, reverse=True)
stock_dict = {}
# assign a score to each stock, you can also change the rule of scoring here.
for i,ele in enumerate(sortedByfactor1):
rank1 = i
rank2 = sortedByfactor2.index(ele)
rank3 = sortedByfactor3.index(ele)
score = sum([rank1*0.4,rank2*0.2,rank3*0.4])
stock_dict[ele] = score
# sort the stocks by their scores
self.sorted_stock = sorted(stock_dict.items(), key=lambda d:d[1],reverse=False)
sorted_symbol = [x[0] for x in self.sorted_stock]
self.sorted_stock = sorted(stock_dict.items(), key=lambda d:d[1],reverse=True)
#
self.sorted_symbol = [self.sorted_stock[i][0] for i in range(len(self.sorted_stock))]
topFine = self.sorted_symbol[:self.__numberOfSymbolsFine]
self.XLVstocks = [i.Symbol for i in topFine]
# for symbol in self.manually_selected:
# self.XLVstocks.append(symbol)
return self.XLVstocks
def XLCfine(self, fine):
filtered_fine = [x for x in fine if x.AssetClassification.MorningstarSectorCode == MorningstarSectorCode.CommunicationServices
and (x.ValuationRatios.EVToEBITDA > 0)
and (x.EarningReports.BasicAverageShares.ThreeMonths > 0)
and x.EarningReports.BasicAverageShares.ThreeMonths * (x.EarningReports.BasicEPS.TwelveMonths*x.ValuationRatios.PERatio) > 2e9]
sortedByfactor1 = sorted(filtered_fine, key=lambda x: x.OperationRatios.ROIC.Value, reverse=True)
sortedByfactor2 = sorted(filtered_fine, key=lambda x: x.OperationRatios.LongTermDebtEquityRatio.Value, reverse=True)
sortedByfactor3 = sorted(filtered_fine, key=lambda x: x.ValuationRatios.FCFYield, reverse=True)
stock_dict = {}
# assign a score to each stock, you can also change the rule of scoring here.
for i,ele in enumerate(sortedByfactor1):
rank1 = i
rank2 = sortedByfactor2.index(ele)
rank3 = sortedByfactor3.index(ele)
score = sum([rank1*0.4,rank2*0.2,rank3*0.4])
stock_dict[ele] = score
# sort the stocks by their scores
self.sorted_stock = sorted(stock_dict.items(), key=lambda d:d[1],reverse=False)
sorted_symbol = [x[0] for x in self.sorted_stock]
self.sorted_stock = sorted(stock_dict.items(), key=lambda d:d[1],reverse=True)
#
self.sorted_symbol = [self.sorted_stock[i][0] for i in range(len(self.sorted_stock))]
topFine = self.sorted_symbol[:self.__numberOfSymbolsFine]
self.XLCstocks = [i.Symbol for i in topFine]
# for symbol in self.manually_selected:
# self.XLCstocks.append(symbol)
return self.XLCstocks
def Rebalance(self):
if not self.mom.IsReady or not self.sma.IsReady: return
initial_asset = self.stock if self.mom.Current.Value > 0 else self.bond
if self.init:
self.SetHoldings(initial_asset, .01)
self.init = False
keihist = self.History([self.kei], (int(self.GetParameter("keihist"))))
#keihist = keihist['Value'].unstack(level=0).dropna()
keihistlowt = np.nanpercentile(keihist, 15)
keihistmidt = np.nanpercentile(keihist, 50)
keihisthight = np.nanpercentile(keihist, 90)
kei = self.sma.Current.Value
keimom = self.mom.Current.Value
if (keimom < 0 and kei < keihistmidt and kei > keihistlowt) and not (self.Securities[self.bond].Invested) :
# DECLINE
self.Liquidate()
self.SetHoldings(self.XLP, .01)
for symbol, symbolData in self.XLPsector_symbolDataBySymbol.items():
if not self.Portfolio[symbol].Invested : #and (self.Securities[symbol].Close > symbolData.sma50.Current.Value):
self.SetHoldings(symbol, .1, False, "Buy Signal")
#Stop Loss
# for symbol, symbolData in self.XLPsector_symbolDataBySymbol.items():
# if not (self.Securities[symbol].Close < symbolData.low.Current.Value) and (self.Securities[self.XLB].Invested):
# self.Liquidate()
# self.SetHoldings(self.XLP, .01)
self.SetHoldings(self.bond, .4)
self.Debug("STAPLES {0} >> {1}".format(self.XLP, self.Time))
elif (keimom > 0 and kei < keihistlowt) and not (self.Securities[self.XLB].Invested) :
# RECOVERY
self.Liquidate()
self.SetHoldings(self.XLB, .01)
for symbol, symbolData in self.XLEsector_symbolDataBySymbol.items():
if not self.Portfolio[symbol].Invested : #and (self.Securities[symbol].Close > symbolData.sma50.Current.Value):
self.SetHoldings(symbol, .1, False, "Buy Signal")
#XLB
for symbol, symbolData in self.XLBsector_symbolDataBySymbol.items():
if not self.Portfolio[symbol].Invested : #and (self.Securities[symbol].Close > symbolData.sma50.Current.Value):
self.SetHoldings(symbol, .1, False, "Buy Signal")
#XLY
for symbol, symbolData in self.XLYsector_symbolDataBySymbol.items():
if not self.Portfolio[symbol].Invested : #and (self.Securities[symbol].Close > symbolData.sma50.Current.Value):
self.SetHoldings(symbol, .1, False, "Buy Signal")
#Stop Loss
# for symbol, symbolData in self.XLBsector_symbolDataBySymbol.items():
# if not (self.Securities[symbol].Close < symbolData.low.Current.Value) and (self.Securities[self.XLB].Invested):
# self.Liquidate()
# self.SetHoldings(self.XLB, .01)
self.Debug("MATERIALS {0} >> {1}".format(self.XLB, self.Time))
elif (keimom > 0 and kei > keihistlowt and kei < keihistmidt) and not (self.Securities[self.XLE].Invested) :
# EARLY
self.Liquidate()
self.SetHoldings(self.XLE, .01)
#XLF
for symbol, symbolData in self.XLFsector_symbolDataBySymbol.items():
if not self.Portfolio[symbol].Invested : #and (self.Securities[symbol].Close > symbolData.sma50.Current.Value):
self.SetHoldings(symbol, .1, False, "Buy Signal")
#XLI
for symbol, symbolData in self.XLIsector_symbolDataBySymbol.items():
if not self.Portfolio[symbol].Invested : #and (self.Securities[symbol].Close > symbolData.sma50.Current.Value):
self.SetHoldings(symbol, .1, False, "Buy Signal")
#XLE
for symbol, symbolData in self.XLEsector_symbolDataBySymbol.items():
if not self.Portfolio[symbol].Invested : #and (self.Securities[symbol].Close > symbolData.sma50.Current.Value):
self.SetHoldings(symbol, .1, False, "Buy Signal")
#Stop Loss
# for symbol, symbolData in self.XLEstocks.items():
# if not (self.Securities[symbol].Close < symbolData.low.Current.Value) and (self.Securities[self.XLE].Invested):
# self.Liquidate()
# self.SetHoldings(self.XLE, .01)
self.Debug("ENERGY {0} >> {1}".format(self.XLE, self.Time))
elif (keimom > 0 and kei > keihistmidt and kei < keihisthight) and not (self.Securities[self.XLU].Invested) :
# REBOUND
self.Liquidate()
#self.SetHoldings(self.XLK, .5)
self.SetHoldings(self.XLU, .01)
#XLU
for symbol, symbolData in self.XLUsector_symbolDataBySymbol.items():
if not self.Portfolio[symbol].Invested : #and (self.Securities[symbol].Close > symbolData.sma50.Current.Value):
self.SetHoldings(symbol, .2, False, "Buy Signal")
#Stop Loss
# for symbol, symbolData in self.XLUsector_symbolDataBySymbol.items():
# if not (self.Securities[symbol].Close < symbolData.low.Current.Value) and (self.Securities[self.XLU].Invested):
# self.Liquidate()
# self.SetHoldings(self.XLU, .01)
self.Debug("UTILITIES {0} >> {1}".format(self.XLU, self.Time))
elif (keimom < 0 and kei < keihisthight and kei > keihistmidt) and not (self.Securities[self.XLK].Invested) :
# LATE
self.Liquidate()
self.SetHoldings(self.XLK, .01)
for symbol, symbolData in self.XLKsector_symbolDataBySymbol.items():
if not self.Portfolio[symbol].Invested and (self.Securities[symbol].Close > symbolData.sma50.Current.Value) :
self.SetHoldings(symbol, .1, False, "Buy Signal")
#self.SetHoldings(self.XLV, .5)
for symbol, symbolData in self.XLVsector_symbolDataBySymbol.items():
if not self.Portfolio[symbol].Invested and (self.Securities[symbol].Close > symbolData.sma50.Current.Value) :
self.SetHoldings(symbol, .1, False, "Buy Signal")
self.Debug("INFO TECH {0} >> {1}".format(self.XLK, self.Time))
#Stop Loss
# for symbol, symbolData in self.XLKsector_symbolDataBySymbol.items():
# if not (self.Securities[symbol].Close < symbolData.low.Current.Value) and (self.Securities[self.XLK].Invested):
# self.Liquidate()
# self.SetHoldings(self.XLK, .01)
elif (keimom < 0 and kei < 100 and not self.Securities[self.bond].Invested):
self.Liquidate()
self.SetHoldings(self.bond, .5)
self.Plot("LeadInd", "SMA(LeadInd)", self.sma.Current.Value)
self.Plot("LeadInd", "THRESHOLD", 100)
self.Plot("MOMP", "MOMP(LeadInd)", self.mom.Current.Value)
self.Plot("MOMP", "THRESHOLD", 0)
def buySignals(self):
if self.trade == False:
return
## EARLY - XLE
for symbol, symbolData in self.XLEsector_symbolDataBySymbol.items():
if self.Portfolio[self.XLE].Invested:
self.SetHoldings(symbol, .15, False, "Buy Signal")
for symbol, symbolData in self.XLFsector_symbolDataBySymbol.items():
if self.Portfolio[self.XLE].Invested:
self.SetHoldings(symbol, .15, False, "Buy Signal")
for symbol, symbolData in self.XLIsector_symbolDataBySymbol.items():
if self.Portfolio[self.XLE].Invested:
self.SetHoldings(symbol, .15, False, "Buy Signal")
## Recovery - XLB
for symbol, symbolData in self.XLEsector_symbolDataBySymbol.items():
if self.Portfolio[self.XLB].Invested:
self.SetHoldings(symbol, .2, False, "Buy Signal")
for symbol, symbolData in self.XLBsector_symbolDataBySymbol.items():
if self.Portfolio[self.XLB].Invested:
self.SetHoldings(symbol, .2, False, "Buy Signal")
for symbol, symbolData in self.XLYsector_symbolDataBySymbol.items():
if self.Portfolio[self.XLB].Invested:
self.SetHoldings(symbol, .2, False, "Buy Signal")
## Rebound - XLU
for symbol, symbolData in self.XLUsector_symbolDataBySymbol.items():
if self.Portfolio[self.XLU].Invested:
self.SetHoldings(symbol, .2, False, "Buy Signal")
## Decline - XLP
for symbol, symbolData in self.XLPsector_symbolDataBySymbol.items():
if self.Portfolio[self.XLP].Invested:
self.SetHoldings(symbol, .2, False, "Buy Signal")
## Late - XLK
for symbol, symbolData in self.XLKsector_symbolDataBySymbol.items():
if self.Portfolio[self.XLK].Invested:
self.SetHoldings(symbol, .2, False, "Buy Signal")
for symbol, symbolData in self.XLVsector_symbolDataBySymbol.items():
if self.Portfolio[self.XLK].Invested:
self.SetHoldings(symbol, .2, False, "Buy Signal")
class SelectionData(object):
def __init__(self, symbol, period):
self.symbol = symbol
self.ema = ExponentialMovingAverage(period)
self.is_above_ema = False
self.volume = 0
def update(self, time, price, volume):
self.volume = volume
if self.ema.Update(time, price):
self.is_above_ema = price > ema
class FScore(object):
def __init__(self, netincome, operating_cashflow, roa_current,
roa_past, issued_current, issued_past, grossm_current, grossm_past,
longterm_current, longterm_past, curratio_current, curratio_past,
assetturn_current, assetturn_past):
self.netincome = netincome
self.operating_cashflow = operating_cashflow
self.roa_current = roa_current
self.roa_past = roa_past
self.issued_current = issued_current
self.issued_past = issued_past
self.grossm_current = grossm_current
self.grossm_past = grossm_past
self.longterm_current = longterm_current
self.longterm_past = longterm_past
self.curratio_current = curratio_current
self.curratio_past = curratio_past
self.assetturn_current = assetturn_current
self.assetturn_past = assetturn_past
def ObjectiveScore(self):
fscore = 0
fscore += np.where(self.netincome > 0, 1, 0)
fscore += np.where(self.operating_cashflow > 0, 1, 0)
fscore += np.where(self.roa_current > self.roa_past, 1, 0)
fscore += np.where(self.operating_cashflow > self.roa_current, 1, 0)
fscore += np.where(self.longterm_current <= self.longterm_past, 1, 0)
fscore += np.where(self.curratio_current >= self.curratio_past, 1, 0)
fscore += np.where(self.issued_current <= self.issued_past, 1, 0)
fscore += np.where(self.grossm_current >= self.grossm_past, 1, 0)
fscore += np.where(self.assetturn_current >= self.assetturn_past, 1, 0)
return fscore
class SymbolData:
def __init__(self, symbol, sma7, ema10, sma20, sma50, sma200, ema20, rsi, wilr, wilr_fast, high, midhigh, low, stoplow):
self.Symbol = symbol
self.sma7 = sma7
self.ema10 = ema10
self.sma20 = sma20
self.sma50 = sma50
self.sma200 = sma200
self.ema20 = ema20
self.rsi = rsi
self.wilr = wilr
self.wilr_fast = wilr_fast
self.high = high
self.midhigh = midhigh
self.low = low
self.stoplow = stoplow
# Quandl often doesn't use close columns so need to tell LEAN which is the "value" column.
class QuandlCustomColumns(PythonQuandl):
def __init__(self):
# Define ValueColumnName: cannot be None, Empty or non-existant column name
self.ValueColumnName = "Value"
Louis Szeto
Hi Axist
Please include the below line, since importing QC dependencies will not be autocomplete in IDE 3.0.
Best
Louis
The material on this website is provided for informational purposes only and does not constitute an offer to sell, a solicitation to buy, or a recommendation or endorsement for any security or strategy, nor does it constitute an offer to provide investment advisory services by QuantConnect. In addition, the material offers no opinion with respect to the suitability of any security or specific investment. QuantConnect makes no guarantees as to the accuracy or completeness of the views expressed in the website. The views are subject to change, and may have become unreliable for various reasons, including changes in market conditions or economic circumstances. All investments involve risk, including loss of principal. You should consult with an investment professional before making any investment decisions.
Axist
The material on this website is provided for informational purposes only and does not constitute an offer to sell, a solicitation to buy, or a recommendation or endorsement for any security or strategy, nor does it constitute an offer to provide investment advisory services by QuantConnect. In addition, the material offers no opinion with respect to the suitability of any security or specific investment. QuantConnect makes no guarantees as to the accuracy or completeness of the views expressed in the website. The views are subject to change, and may have become unreliable for various reasons, including changes in market conditions or economic circumstances. All investments involve risk, including loss of principal. You should consult with an investment professional before making any investment decisions.
To unlock posting to the community forums please complete at least 30% of Boot Camp.
You can continue your Boot Camp training progress from the terminal. We hope to see you in the community soon!