Optimization
Parameters
Introduction
Parameters are project variables that your algorithm uses to define the value of internal variables like indicator arguments or the length of lookback windows.
Parameters are stored outside of your algorithm code, but we inject the values of the parameters into your algorithm when you launch an optimization job. The optimizer adjusts the value of your project parameters across a range and step size that you define to minimize or maximize an objective function. To optimize some parameters, add some parameters to your project and add the GetParameter
get_parameter
method to your code files.
Set Parameters
Algorithm parameters are hard-coded values for variables in your project that are set outside of the code files. Add parameters to your projects to remove hard-coded values from your code files and to perform parameter optimizations. You can add parameters, set default parameter values, and remove parameters from your projects.
Add Parameters
Follow these steps to add an algorithm parameter to a project:
- Open the project.
- In the Project panel, click .
- Enter the parameter name.
- Enter the default value.
- Click .
The parameter name must be unique in the project.
To get the parameter values into your algorithm, see Get Parameters.
Set Default Parameter Values
Follow these steps to set the default value of an algorithm parameter in a project:
- Open the project.
- In the Project panel, hover over the algorithm parameter and then click the pencil icon that appears.
- Enter a default value for the parameter and then click .
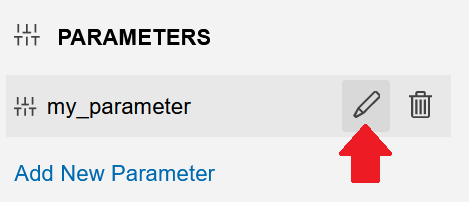
The Project panel displays the default parameter value next to the parameter name.
Delete Parameters
Follow these steps to delete an algorithm parameter in a project:
- Open the project.
- In the Project panel, hover over the algorithm parameter and then click the trash can icon that appears.
- Remove the
GetParameter
calls that were associated with the parameter from your code files.
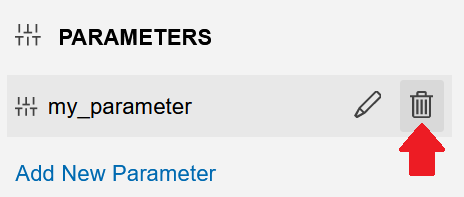
Get Parameters
To get the parameter values from the Project panel into your algorithm, see Get Parameters.
Number of Parameters
The cloud optimizer can optimize up to three parameters. There are several reasons for this quota. First, the optimizer only supports the grid search strategy, which is very inefficient. This strategy tests every permutation of parameter values, so the number of backtests that the optimization job must run explodes as you add more parameters. Second, the parameter charts that display the optimization results are limited to three dimensions. Third, if you optimize with many variables, it increases the likelihood of overfitting to historical data.
To optimize more than three parameters, run local optimizations with the CLI.