Popular Libraries
Keras
Create Subscriptions
In the Initialize
initialize
method, subscribe to some data so you can train the keras
model and make predictions.
self._symbol = self.add_equity("SPY", Resolution.DAILY).symbol
Build Models
In this example, build a neural-network regression model that uses the following features and labels:
Data Category | Description |
---|---|
Features | Daily percent change of the open, high, low, close, and volume of the SPY over the last 5 days |
Labels | Daily percent return of the SPY over the next day |
The following image shows the time difference between the features and labels:
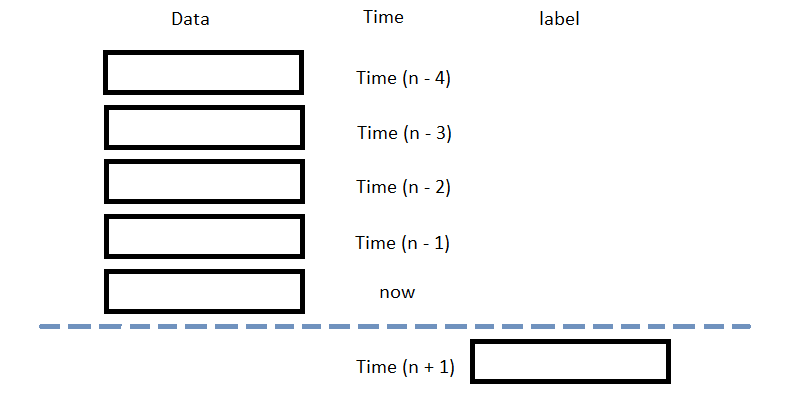
Follow the below steps to build the model:
- In the
Initialize
initialize
method, create aSequential
object with several layers. - Call the
compile
method with a loss function, an optimizer, and a list of metrics to monitor.
self.model = Sequential([Dense(10, input_shape=(5,5), activation='relu'), Dense(10, activation='relu'), Flatten(), Dense(1)])
Set the input_shape
of the first layer to (5, 5)
because each sample contains the percent change of 5 factors (percent change of the open, high, low, close, and volume) over the previous 5 days. Call the Flatten
constructor because the input is 2-dimensional but the output is just a single value.
self.model.compile(loss='mse', optimizer=Adam(), metrics=['mae', 'mse'])
Train Models
You can train the model at the beginning of your algorithm and you can periodically re-train it as the algorithm executes.
Warm Up Training Data
You need historical data to initially train the model at the start of your algorithm. To get the initial training data, in the Initialize
initialize
method, make a history request.
training_length = 252*2 self.training_data = RollingWindow[TradeBar](training_length) history = self.history[TradeBar](self._symbol, training_length, Resolution.DAILY) for trade_bar in history: self.training_data.add(trade_bar)
Define a Training Method
To train the model, define a method that fits the model with the training data.
def get_features_and_labels(self, n_steps=5): training_df = self.pandas_converter.get_data_frame[TradeBar](list(self.training_data)[::-1]) daily_pct_change = training_df.pct_change().dropna() features = [] labels = [] for i in range(len(daily_pct_change)-n_steps): features.append(daily_pct_change.iloc[i:i+n_steps].values) labels.append(daily_pct_change['close'].iloc[i+n_steps]) features = np.array(features) labels = np.array(labels) return features, labels def my_training_method(self): features, labels = self.get_features_and_labels() self.model.fit(features, labels, epochs=5)
Set Training Schedule
To train the model at the beginning of your algorithm, in the Initialize
initialize
method, call the Train
train
method.
self.train(self.my_training_method)
To periodically re-train the model as your algorithm executes, in the Initialize
initialize
method, call the Train
train
method as a Scheduled Event.
# Train the model every Sunday at 8:00 AM self.train(self.date_rules.every(DayOfWeek.SUNDAY), self.time_rules.at(8, 0), self.my_training_method)
Update Training Data
To update the training data as the algorithm executes, in the OnData
on_data
method, add the current TradeBar
to the RollingWindow
that holds the training data.
def on_data(self, slice: Slice) -> None: if self._symbol in slice.Bars: self.training_data.Add(slice.Bars[self._symbol])
Predict Labels
To predict the labels of new data, in the OnData
on_data
method, get the most recent set of features and then call the predict
method.
features, _ = self.get_features_and_labels() features = features[-1].reshape(1, 5, 5) prediction = float(self.model.predict(features)[-1])
You can use the label prediction to place orders.
if prediction > 0: self.SetHoldings(self._symbol, 1) elif prediction < 0: self.SetHoldings(self._symbol, -1)
Save Models
Follow these steps to save keras
models into the Object Store:
- Set the key name you want to store the model under in the Object Store.
- Call the
GetFilePath
get_file_path
method with the key. - Call the
save
method with the file path.
The key must end with a .keras extension for the native Keras format (recommended) or a .h5 extension.
model_key = "model.keras"
file_name = self.object_store.get_file_path(model_key)
This method returns the file path where the model will be stored.
self.model.save(file_name)
Load Models
You can load and trade with pre-trained keras
models that you saved in the Object Store. To load a keras
model from the Object Store, in the Initialize
initialize
method, get the file path to the saved model and then call the load_model
method.
def initialize(self) -> None: if self.object_store.contains_key(model_key): file_name = self.object_store.get_file_path(model_key) self.model = load_model(file_name)
The ContainsKey
contains_key
method returns a boolean that represents if the model_key
is in the Object Store. If the Object Store does not contain the model_key
, save the model using the model_key
before you proceed.